Robotics PhD at Stanford University
I am a researcher with 8+ years of experience in robotics and AI. My expertise lies in the intersection between robot tactile perception, dynamics and robot control. I leverage a diverse set of skills from machine learning to hardware design to come up with creative technical solutions.
I obtained my PhD from Stanford University where I was part of the Biomimetric and Dexterous Manipulation Lab, advised by Prof. Mark Cutkosky. I was a Research Intern at the NVIDIA Seattle Robotics Lab where I investigated methods to transfer robot skills learned in simulation to the real world (sim2real) by making simulator dynamics more realistic. Prior to my PhD, I was a Robotics System Engineer at Flexiv Robotics Inc. developing the mechatronics and controls of a 7 degrees-of-freedom torque-controlled robot arm for industrial task automation. I completed my undergraduate in Electrical Engineering and Computer Science at UC Berkeley with a focus in mechatronics and signals and systems.
Contact:
mlinyang@stanford.edu
Google Scholar
Follow @michaelv03
Latest Research
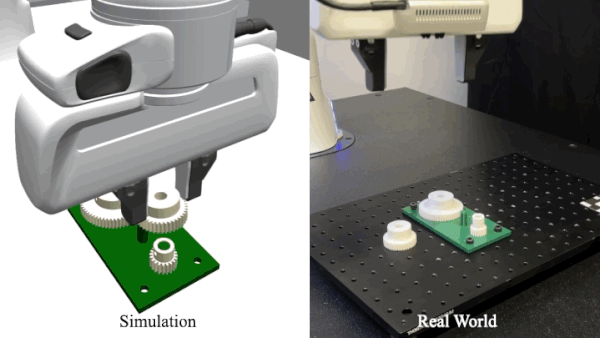
IndustReal: Transferring Contact-Rich Assembly Tasks from Simulation to Reality
Robotic assembly is a longstanding challenge, requiring contact-rich interaction and high precision and accuracy. Many applications also require adaptivity to diverse parts, poses, and environments, as well as low cycle times. In other areas of robotics, simulation is a powerful tool to develop algorithms, generate datasets, and train agents. However, simulation has had a more limited impact on assembly. We present IndustReal, a set of algorithms, systems, and tools that solve assembly tasks in simulation with reinforcement learning (RL) and successfully achieve policy transfer to the real world. Specifically, we propose 1) simulation-aware policy updates, 2) signed-distance-field rewards, and 3) sampling-based curricula for robotic RL agents. We use these algorithms to enable robots to solve contact-rich pick, place, and insertion tasks in simulation. We then propose 4) a policy-level action integrator to minimize error at policy deployment time. We build and demonstrate a real-world robotic assembly system that uses the trained policies and action integrator to achieve repeatable performance in the real world. Finally, we present hardware and software tools that allow other researchers to fully reproduce our system and results.
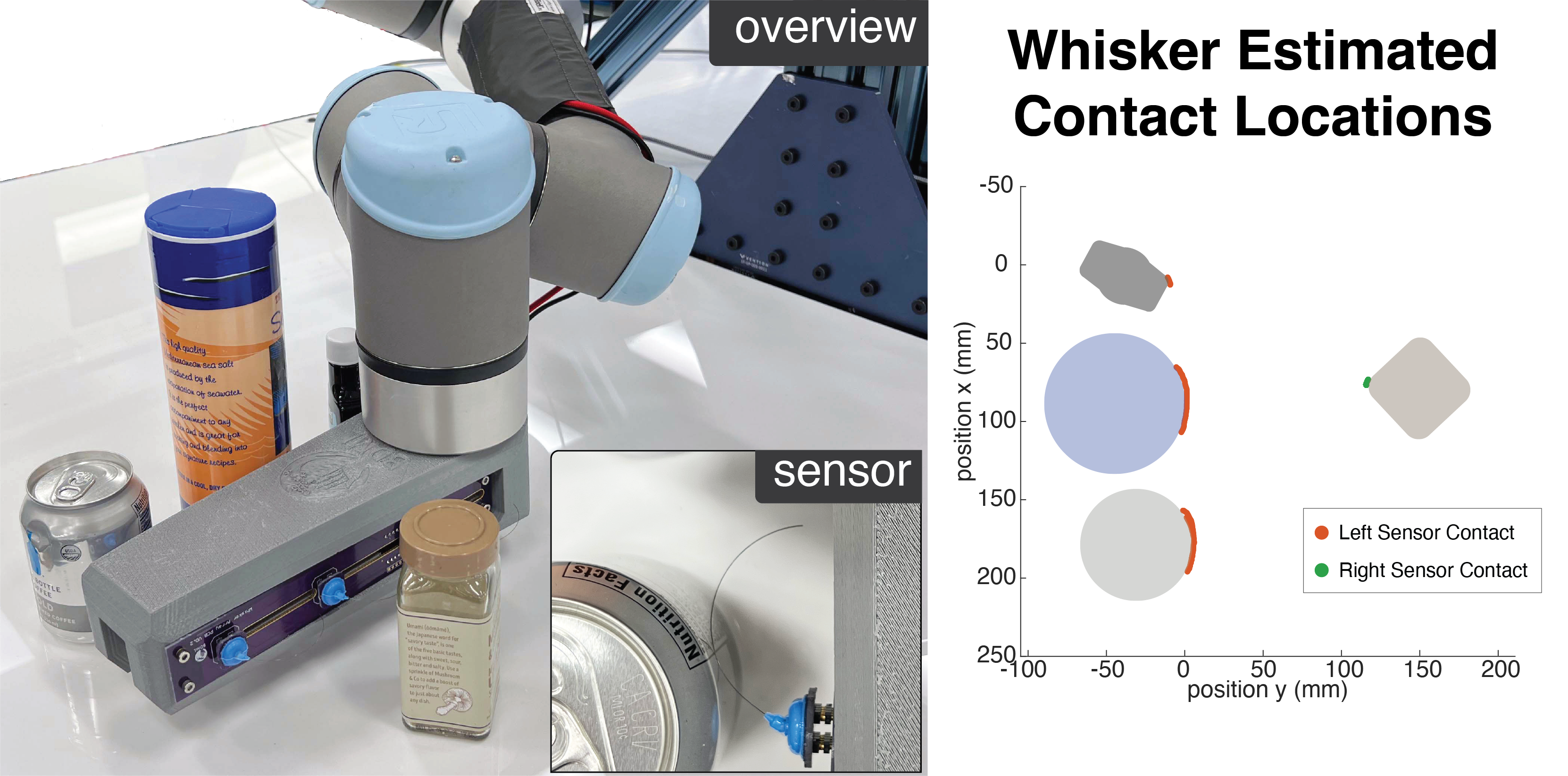
Whisker-Inspired Tactile Sensing for Contact Localization on Robot Manipulators
This work presents the design and modelling of whisker-inspired sensors that attach to the surface of a robot manipulator to sense its surrounding through light contacts. We obtain a sensor model using a calibration process that applies to straight and curved whiskers. We then propose a sensing algorithm using Bayesian filtering to localize contact points. The algorithm combines the accurate proprioceptive sensing of the robot and sensor readings from the deflections of the whiskers. Our results show that our algorithm is able to track contact points with sub-millimeter accuracy, outperforming a baseline method. Finally, we demonstrate our sensor and perception method in a real-world system where a robot moves in between free-standing objects and uses the whisker sensors to track contacts tracing object contours.
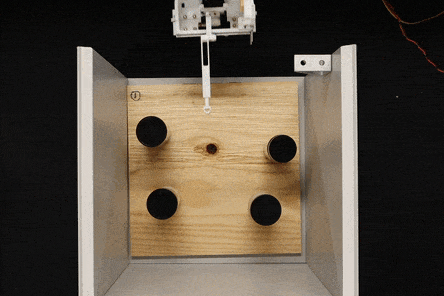
Exploratory Hand: Leveraging Safe Contact to Facilitate Manipulation in Cluttered Spaces
We present a new gripper and exploration approach that uses an exploratory finger with very low reflected inertia for probing and grasping objects quickly and safely in unstructured environments. Equipped with sensing and force control, the gripper allows a robot to leverage contact information to accurately estimate object location through a particle filtering algorithm and also grasp objects with location uncertainty based on a contact-first approach. This publication is still under review so it is not yet available.
A Stretchable Tactile Sleeve for Reaching into Cluttered Spaces
A highly conformable stretchable sensory skin made entirely of soft components. The skin uses pneumatic taxels and stretchable channels to conduct pressure signals to off-board MEMs pressure sensors. The skin is able to resolve forces down to 0.01N and responds to vibrations up to 200 Hz. We apply the skin to a 2 degree-of-freedom robotic wrist with intersecting axes for manipulation in constrained spaces, and show that it has sufficient sensitivity and bandwidth to detect the onset of sliding as the robot contacts objects. We demonstrate the skin in object acquisition tasks in a tightly constrained environment for which extraneous contacts are unavoidable.